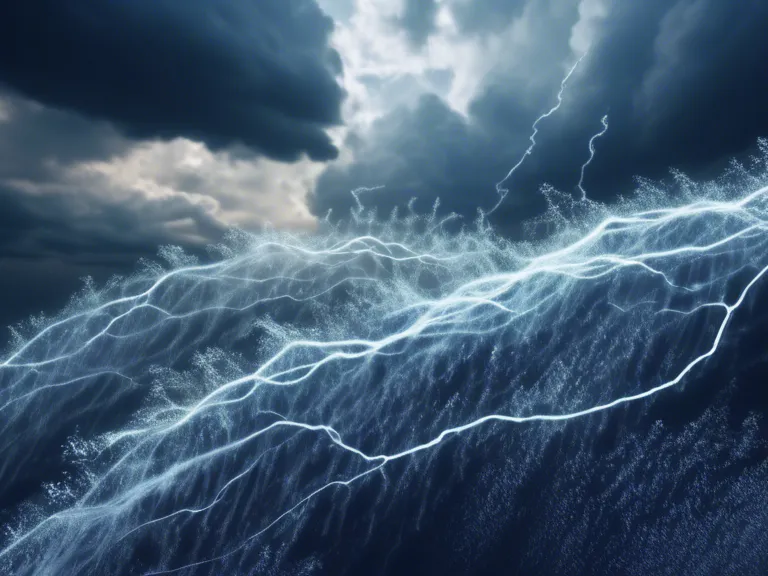
Introduction
Weather forecasting has always been a challenging task due to the complex and dynamic nature of the Earth's atmosphere. However, with the advancements in technology, especially in the field of machine learning, there have been significant improvements in weather forecasting accuracy and lead time. In this article, we will explore how machine learning is revolutionizing weather forecasting and the key advancements it has brought to this critical field.
Machine Learning in Weather Forecasting
Data Collection and Processing
One of the key areas where machine learning has made a significant impact in weather forecasting is in data collection and processing. Machine learning algorithms can analyze vast amounts of data from various sources such as satellites, weather stations, and sensors in real-time. This enables meteorologists to have access to more accurate and timely data for their forecasts.
Model Development
Machine learning algorithms are also being used to develop more sophisticated and accurate weather prediction models. These models can take into account a wide range of variables and factors, such as temperature, humidity, wind speed, and air pressure, to provide more reliable forecasts. Machine learning algorithms can continuously learn and improve from new data, leading to more accurate predictions over time.
Extreme Weather Events Prediction
Machine learning is also being used to predict extreme weather events, such as hurricanes, tornadoes, and heavy rainfall. By analyzing historical weather data and patterns, machine learning algorithms can identify potential triggers for these events and provide early warnings to help communities prepare and mitigate the impact of such disasters.
Benefits of Machine Learning in Weather Forecasting
Improved Accuracy
One of the most significant benefits of using machine learning in weather forecasting is the improved accuracy of predictions. Machine learning algorithms can analyze complex patterns and relationships in data that traditional forecasting methods may overlook, leading to more precise and reliable forecasts.
Faster Forecasting
Machine learning algorithms can process large amounts of data quickly, allowing meteorologists to generate forecasts in real-time. This rapid processing speed is crucial for predicting fast-changing weather conditions and providing timely warnings to the public.
Enhanced Resilience
By providing more accurate and timely weather forecasts, machine learning can help communities and emergency responders better prepare for extreme weather events. This enhanced resilience can save lives and reduce the economic impact of disasters.
Challenges and Future Directions
While machine learning has brought significant advancements to weather forecasting, there are still challenges that need to be addressed. One of the key challenges is the need for high-quality data to train machine learning models effectively. Additionally, ensuring the interpretability and transparency of machine learning algorithms in weather forecasting is crucial to building trust with stakeholders and the public.
In the future, we can expect further advancements in machine learning techniques for weather forecasting, such as the integration of artificial intelligence and deep learning to improve model performance. Collaborations between meteorologists, data scientists, and technology experts will be essential to drive innovation in this critical field.
Conclusion
Machine learning has transformed weather forecasting by enabling more accurate predictions, faster forecasting, and enhanced resilience to extreme weather events. As technology continues to evolve, we can expect even more significant advancements in the field of weather forecasting, ultimately benefiting society as a whole. By harnessing the power of machine learning, we can better understand and predict the complexities of the Earth's atmosphere, leading to a safer and more prepared world.