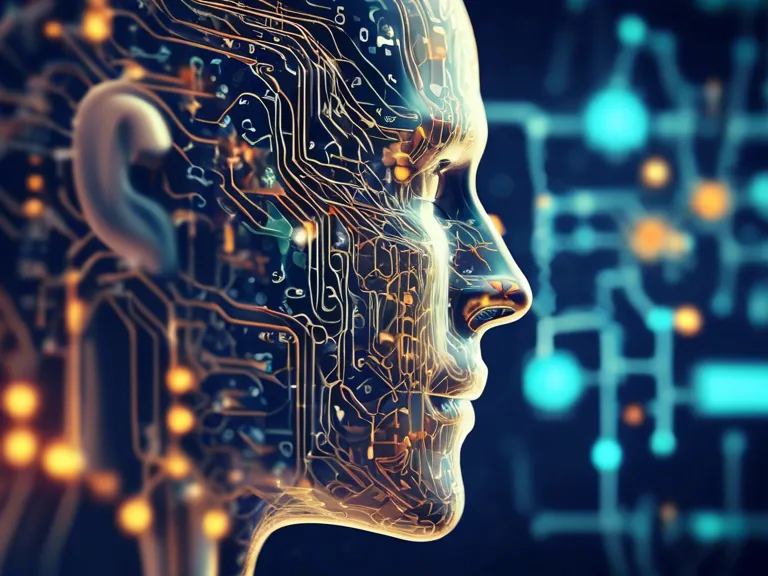
Machine learning has revolutionized the way businesses operate by utilizing data-driven algorithms to make predictions and decisions. In today's fast-paced business environment, organizations are constantly seeking ways to maximize profits and gain a competitive edge. One of the most effective ways to achieve this is through the application of machine learning techniques. By leveraging machine learning applications, businesses can uncover valuable insights from their data, optimize processes, and drive revenue growth.
Understanding Machine Learning
Machine learning is a branch of artificial intelligence that enables computers to learn from data without being explicitly programmed. It involves the development of algorithms and models that can analyze and interpret large datasets to identify patterns, make predictions, and generate insights.
Leveraging Machine Learning for Profit Maximization
1. Predictive Analytics
Predictive analytics is a powerful application of machine learning that enables businesses to forecast future outcomes based on historical data. By analyzing customer behavior, market trends, and other relevant factors, organizations can make informed decisions to optimize pricing strategies, inventory management, and customer targeting.
2. Customer Segmentation
Machine learning algorithms can segment customers based on their preferences, behavior, and purchasing patterns. By understanding the different segments within their customer base, businesses can tailor marketing campaigns, product recommendations, and pricing strategies to maximize customer satisfaction and loyalty.
3. Fraud Detection
Fraud detection is a critical application of machine learning for businesses in various industries, including finance, e-commerce, and healthcare. Machine learning models can analyze transaction data in real-time to identify suspicious activities and prevent fraudulent behavior, ultimately protecting businesses from financial losses.
4. Demand Forecasting
Machine learning can help businesses accurately forecast demand for their products or services by analyzing historical sales data, seasonality trends, and external factors such as economic indicators or weather conditions. By optimizing inventory levels and production schedules, organizations can reduce costs and maximize profits.
Implementing Machine Learning Applications
1. Data Collection and Preparation
The first step in implementing machine learning applications is to gather and clean relevant data from various sources. This involves identifying key variables, removing outliers, and ensuring data quality before feeding it into machine learning algorithms.
2. Model Development and Training
Once the data is prepared, businesses can develop machine learning models using techniques such as regression, classification, or clustering. These models are trained on historical data to learn patterns and make predictions that can drive decision-making processes.
3. Model Evaluation and Deployment
After training the models, businesses must evaluate their performance using metrics such as accuracy, precision, and recall. Once satisfied with the model's performance, organizations can deploy it into production systems to generate insights and drive profit-maximizing strategies.
Conclusion
Machine learning applications have the potential to revolutionize the way businesses operate and drive significant profits. By leveraging predictive analytics, customer segmentation, fraud detection, and demand forecasting, organizations can optimize processes, reduce costs, and increase revenue. Implementing machine learning applications requires a strategic approach to data collection, model development, and deployment. By embracing machine learning technologies, businesses can stay ahead of the competition and maximize profits in today's data-driven economy.