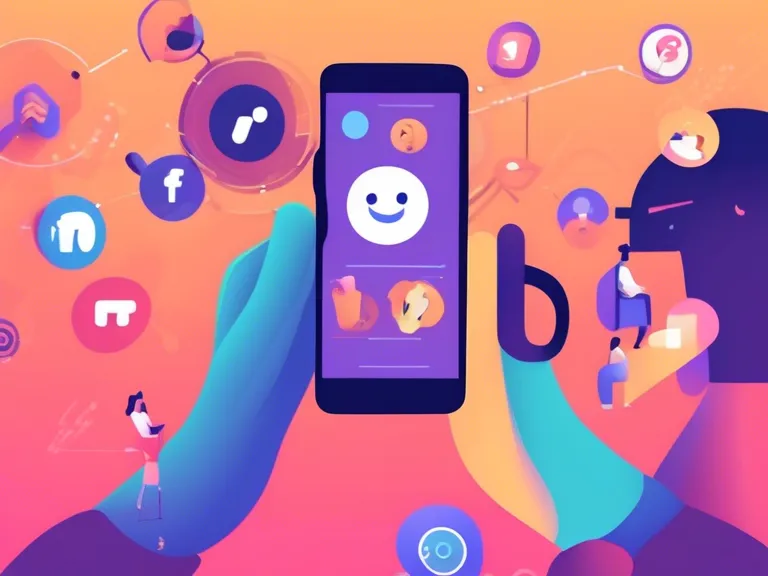
Introduction
Machine learning techniques have revolutionized the way we analyze and understand human behavior on social media platforms. In recent years, TikTok has emerged as one of the most popular social media platforms, with millions of users creating and sharing content daily. Analyzing TikTokers' behavior using machine learning algorithms can provide valuable insights into user preferences, trends, and engagement patterns.
Data Collection
The first step in analyzing TikTokers' behavior using machine learning techniques is data collection. This involves gathering data from the TikTok platform, such as user interactions, engagement metrics, content preferences, and demographic information. Data can be collected using APIs provided by TikTok or through web scraping techniques.
Data Preprocessing
Once the data is collected, it needs to be preprocessed to ensure its quality and relevance for analysis. Data preprocessing tasks may include cleaning the data, handling missing values, encoding categorical variables, and scaling numerical features. This step is crucial for preparing the data for machine learning algorithms.
Feature Engineering
Feature engineering is an important step in analyzing TikTokers' behavior. It involves selecting and creating relevant features from the dataset that can be used to train machine learning models. Features such as user engagement history, content type, posting frequency, and user demographics can provide valuable insights into user behavior on TikTok.
Machine Learning Models
There are several machine learning models that can be used to analyze TikTokers' behavior. These include clustering algorithms for identifying user segments, classification algorithms for predicting user preferences, and regression algorithms for analyzing engagement patterns. Popular machine learning algorithms such as k-means clustering, decision trees, random forests, and neural networks can be applied to the data for analysis.
Sentiment Analysis
Sentiment analysis is a powerful machine learning technique that can be used to analyze TikTokers' behavior based on their emotions and sentiments expressed in comments and interactions. By analyzing the sentiment of user comments and interactions, marketers and content creators can gain insights into user preferences, trends, and engagement patterns.
Recommendation Systems
Machine learning-based recommendation systems can be used to analyze TikTokers' behavior by recommending personalized content based on user preferences and past interactions. These systems use collaborative filtering and content-based filtering techniques to suggest relevant content to users, increasing engagement and user satisfaction on the platform.
Conclusion
Machine learning techniques offer a powerful way to analyze TikTokers' behavior on the popular social media platform TikTok. By leveraging data collection, preprocessing, feature engineering, and machine learning models, marketers and content creators can gain valuable insights into user preferences, trends, and engagement patterns. Sentiment analysis and recommendation systems further enhance the analysis by providing insights into user emotions and personalized content recommendations. Overall, machine learning techniques play a crucial role in understanding and optimizing user behavior on TikTok.